A Treasury Department report assessing the use of artificial intelligence tools by the financial sector identified a “growing capability gap” in in-house AI use between large and small financial institutions.
The report, released Wednesday by the Treasury’s Office of Cybersecurity and Critical Infrastructure Protection (OCCIP), identified “significant opportunities and challenges that AI presents to the security and resiliency of the financial services sector. The report outlines a series of next steps to address immediate AI-related operational risk, cybersecurity, and fraud challenges,” according to a press release.
The report was written in response to President Joe Biden’s executive order on the trustworthy development and use of AI, issued in October. The OCCIP conducted in-depth interviews with 42 financial services and technology-related companies, ranging from global systemically important financial institutions to local banks and credit unions.
The report said the capability gap for in-house AI tool use between large and small financial institutions is driven by a lack of internal data resources at small institutions, which prevents them from adequately training AI models.
“Additionally, financial institutions that have already migrated to the cloud may have an advantage when it comes to leveraging AI systems in a safe and secure manner,” the agency’s release said.
The gap is particularly notable regarding training AI models in fraud detection, as large institutions have more historical data with which to work. There is insufficient data sharing among firms, the Treasury said, and smaller institutions “generally lack sufficient internal data and expertise to build their own anti-fraud AI models.”
On the regulatory front, the report suggested best practices for data supply chain mapping should be implemented, “to ensure that models are using accurate and reliable data and that privacy and safety are considered,” so financial institutions know where their data is and how it is being used.
The report recommended a standardized description of AI tools might also be necessary, with “nutrition labels” for vendor-provided AI systems and data providers.
“These ‘nutrition labels’ would clearly identify what data was used to train the model, where the data originated, and how any data submitted to the model is being used,” the Treasury said.
A common AI lexicon might also become necessary so the specific capabilities of AI tools could be better described to regulators, other financial institutions, and the public.
The report highlighted how the National Institute of Standards and Technology’s AI Risk Management Framework could be expanded and tailored “to include more applicable content on AI governance and risk management related to the financial services sector.”
Another problem with some AI models is explainability, in that financial institutions cannot adequately explain to regulators and the public how AI-generated decisions were made inside a so-called “black box.”
“The sector would benefit from additional research and development on explainability solutions for black-box systems like generative AI, considering the data used to train the models and the outputs and robust testing and auditing of these models,” the Treasury said.
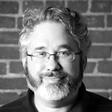
No comments yet